Prediksi kekasaran permukaan baja S45C terhadap parameter pemesinan dan getaran pada proses bubut menggunakan metode artificial neural network
Abstract
Based on machining characteristics, this study gives surface roughness modeling for machine parts. The artificial models used the Artificial Neural Network (ANN) modeling approach and multivariable regression analysis were used to create the prediction model. S45C steel was one of the materials utilized in this research. With a depth of cut 0.5 mm, the parameters are spindle (n) of 165, 330, 585, and 1170 rpm and feed (f) of 0.2 mm/rev. Utilizing TIBCO software, surface roughness values will be predicted. Equations derived from multivariable linear regression serve as the study's findings. At 1170 rpm spindle rotation and 0.5 mm of cut depth, the lowest surface roughness measurement of 1.114 (μm) was recorded. At spindle speed 585 and a cut depth of 2.0 mm, a roughness value of 2.999 (μm) was recorded as the maximum value. Roughness rises at spindle speeds between 585 and 900 rpm when cutting at shallower depths. The third modeling had the smallest error value, which was 11.21%, and surface roughness value using an artificial neural network with five simple multi-layer models.
Keywords
Full Text:
PDFReferences
Amin, A.N., Patwari, A.U., Sharulhazrin, M.S., Hafizuddin, I., Investigation of e_ect of chatter amplitude onsurface roughness during end milling of medium carbon steel, Proc. 2010 Int. Conf. Ind. Eng. Oper. Manag, 127-131, 2010.
Arizmendi, M., Campa, F.J., Fernández, J., De Lacalle, L.L., Gil, A., Bilbao, E., Lamikiz, A., Model for surfacetopography prediction in peripheral milling considering tool vibration, CIRP Ann. Manuf. Technol, 58, 93-96, 2009.
Asiltürk, I., Çunka¸s, M., Modeling and prediction of surface roughness in turning operations using artificialneural network and multiple regression method, Expert. Syst. Appl, 38, 5826-5832, 2011.
Benardos, P.G., Vosniakos, G., Predicting surface roughness in machining: A review, Int. J. Mach. Tools Manuf, 43, 833-844, 2003.
Bhogal, S.S., Sindhu, C., Dhami, S.S., Pabla, B.S., Minimization of surface roughness and tool vibration in CNC milling operation, J. Optim, 2015, 1-13, 2015.
David, C., Sagris, D., Stergianni, E., Tsiafis, C., Tsiafis, I., Experimental analysis of the e_ect of vibration phenomena on workpiece topomorphy due to cutter runout in end-milling process, Machines, 6, 27, 2018.
Stephenson, D.A., Agapiou, J.S., Metal, Cutting Theory and Practice; CRC Press: Boca Raton, FL, USA, 2016.
Qadri, M.O., Namazi, H., Fractal-based analysis of the relation between surface finish and machine vibrationin milling operation, Fluct. Noise Lett, 19, 2050006, 2020.
Zahoor, S., Mufti, N.A., Saleem, M.Q., Mughal, M.P., Qureshi, M.A.M., E_ect of machine tool’s spindle forced vibrations on surface roughness, dimensional accuracy, and tool wear in vertical milling of AISI P20, Int. J. Adv. Manuf. Tech, 89, 3671-3679, 2017.
DOI: https://doi.org/10.29303/dtm.v13i1.605
Refbacks
- There are currently no refbacks.
Copyright (c) 2023

This work is licensed under a Creative Commons Attribution-NonCommercial 4.0 International License.
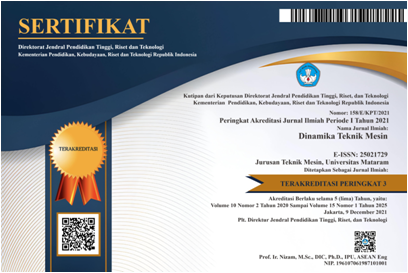